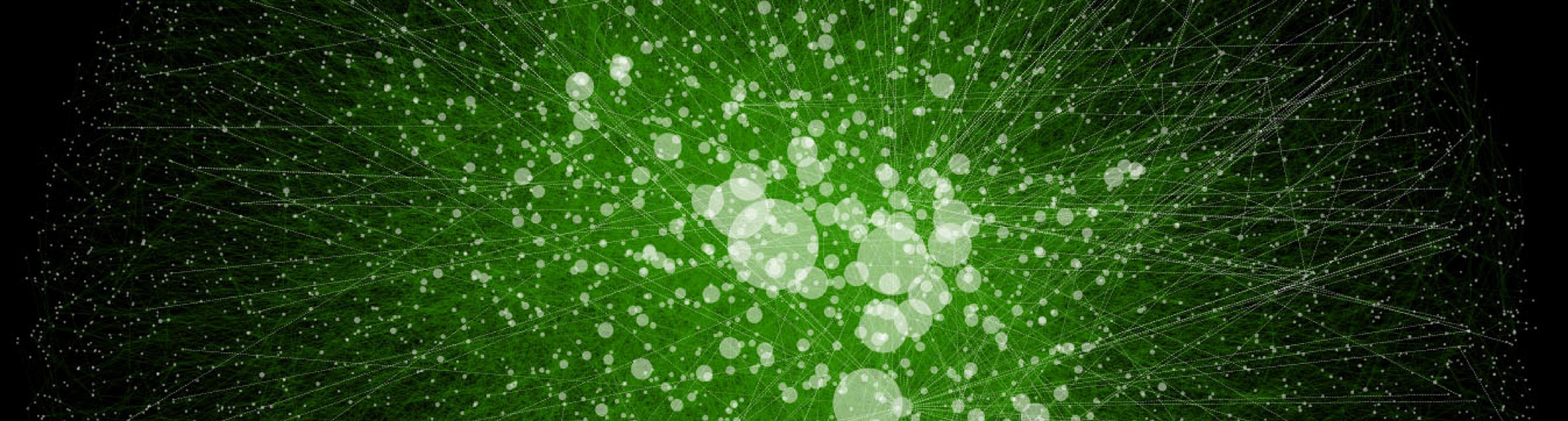
Biomedical Statistics and Informatics
The Division of Biomedical Statistics & Informatics in the UCSF Department of Neurosurgery is involved in a variety of projects related to brain tumor research.
Brain Tumor Center Database
We are developing the user-friendly Brain Tumor Center Database (BTCDB), which provides a streamlined and consolidated research operations network for collecting, sharing and management of clinical and research data centered on patients with gliomas. The platform contains pathology, research genomics, imaging, and epidemiology data from supported projects at UCSF. There is a web interface for data aggregation and exploration (query and filter), data extraction (via pre-defined report templates), and data entry/correction as well as an API for automated interactions. The goal of the Brain Tumor Center database is to leverage clinical and research data to achieve our goal of improved outcomes for patients with brain tumors.
Extent of Resection (EOR) Studies
Glioblastoma EOR Study
We examine the interactive effects of volumetric extent of resection with molecular and clinical factors to develop a new roadmap for cytoreductive surgery. Based on a 20-year retrospective cohort of 850 glioblastoma patients who had initial surgery at UCSF, we employed survival models and recursive partitioning (RPA) to investigate multivariate relationships of overall survival (OS). This is the first study to combine resection of contrast-enhancing and non-enhancing tumor in conjunction with molecular and clinical information in a large single-institution study.
Low-Grade Glioma EOR Study
This study is to test the hypothesis that extent of resection for newly diagnosed WHO II gliomas positively impacts Overall Survival, Progression Free Survival, and time to malignant transformation regardless of tumor genetics.
Immunomethylomics
Gliomas are associated with an immunosuppressive network impacting the tumor microenvironment, bone marrow and the peripheral blood compartments and systemic immune suppressive cells are widely recognized in glioblastoma (GBM) patients. We are developing, testing and using a highly innovative approach called immunomethylomics to measure immunosuppression in glioma patients. Our goal is to develop a non-invasive blood test that helps assess response to glioma therapies and improves understanding of the interaction between the immune system and gliomas. We believe that blood immune profiles may be associated with overall survival and may differentiate true progression from pseudoprogression in GBM patients.
Glioblastoma Precision Medicine Program
Analysis of tumors via next-generation sequencing (NGS) is now routinely used in clinical practice. The glioblastoma precision medicine program is to sequence all newly diagnosed WHO grade IV diffuse gliomas using our UCSF500 NGS panel since December 2017. The UCSF500 Cancer Panel assesses approximately 500 cancer-associated genes for mutations, copy number alterations, and structural rearrangements, including fusions. We review our experience over a 3-year period.
Predicting Tumor Biology Using Multi-modality MRI and Machine Learning
Non-invasive imaging markers of tumor burden, aggressiveness, and effects of therapy are critical in identifying the appropriate therapeutic strategy for an individual patient. The modalities that have shown the most promise in quantifying surrogate markers of malignant progression in patients with gliomas include H-1 MR spectroscopic imaging (MRSI), diffusion-weighted MRI and perfusion-weighted MRI. As recent efforts to standardize acquisition and processing protocols for accumulating multi-institutional datasets are underway, there is the potential for assembling results at a large enough scale to accurately predict the biological behavior of individual tumors. The majority of prior studies have focused on linking parameters from single imaging modalities to outcome or diagnostic classification in relatively small patient populations (<50), have not taken heterogeneity of the lesion into account, especially when defining outcome measures, and have used radiographic response as a surrogate marker of pathology. The overall goal of the project is to assess the clinical value of combining multi-parametric imaging with novel advances in statistical modeling, machine learning, and artificial intelligence to evaluate tumor heterogeneity and identify regions at risk for tumor progression in patients with glioma. Predictive spatial maps of tumor biology will be generated using image-guided tissue samples to link anatomic, physiological and metabolic imaging parameters with histological characteristics. This approach will contribute to patient care by directing tissue sampling to make more accurate diagnoses, improving the characterization of residual disease, assisting in the planning of focal therapy, and detecting malignant progression.